Microbial production of advanced biofuels
Concerns over climate change have necessitated a rethinking of our transportation infrastructure. One possible alternative to carbon-polluting fossil fuels is biofuels produced by engineered microorganisms that use a renewable carbon source. Two biofuels, ethanol and biodiesel, have made inroads in displacing petroleum-based fuels, but their uptake has been limited by the amounts that can be used in conventional engines and by their cost. Advanced biofuels that mimic petroleum-based fuels are not limited by the amounts that can be used in existing transportation infrastructure but have had limited uptake due to costs. In this Review, we discuss engineering metabolic pathways to produce advanced biofuels, challenges with substrate and product toxicity with regard to host microorganisms and methods to engineer tolerance, and the use of functional genomics and machine learning approaches to produce advanced biofuels and prospects for reducing their costs.
This is a preview of subscription content, access via your institution
Access options
Access Nature and 54 other Nature Portfolio journals
Get Nature+, our best-value online-access subscription
cancel any time
Subscribe to this journal
Receive 12 print issues and online access
206,07 € per year
only 17,17 € per issue
Buy this article
- Purchase on SpringerLink
- Instant access to full article PDF
Prices may be subject to local taxes which are calculated during checkout
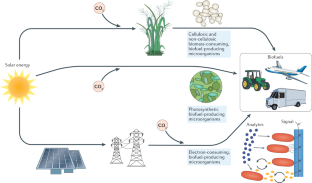
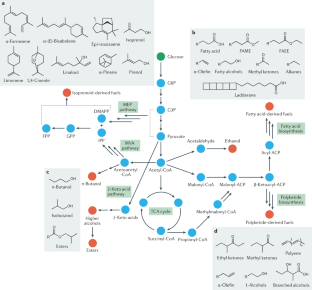
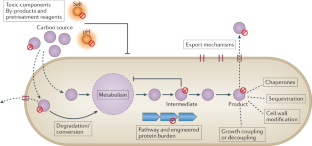
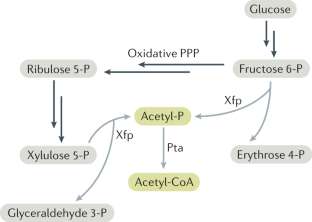
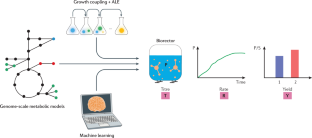
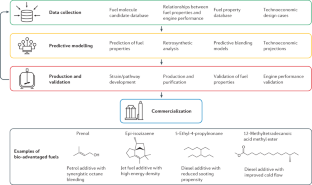
Similar content being viewed by others
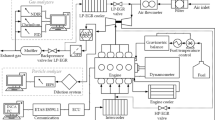
WLTC and real-driving emissions for an autochthonous biofuel from wine-industry waste
Article Open access 06 April 2021
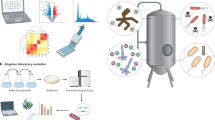
Physiological limitations and opportunities in microbial metabolic engineering
Article 02 August 2021
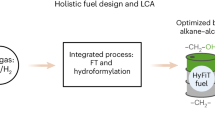
Towards carbon-neutral and clean propulsion in heavy-duty transportation with hydroformylated Fischer–Tropsch fuels
Article Open access 08 July 2024
References
- US Energy Information Administration. International energy outlook 2019: with projections to 2050 (EIA, 2019).
- US Environmental Protection Agency. Sources of greenhouse gas emissions. EPAhttps://www.epa.gov/ghgemissions/sources-greenhouse-gas-emissions (2020).
- Kircher, M. Sustainability of biofuels and renewable chemicals production from biomass. Curr. Opin. Chem. Biol.29, 26–31 (2015). ArticleCASPubMedGoogle Scholar
- Hughes, S. R. & Jones, M. A. in Green Energy to Sustainability: Strategies for Global Industries (eds Vertès, A. A., Qureshi, N., Blaschek, H. P. & Yukawa, H.) 137–156 (Wiley, 2020).
- Liu, Y. et al. Biofuels for a sustainable future. Cell184, 1636–1647 (2021). ArticleCASPubMedGoogle Scholar
- Field, J. L. et al. Robust paths to net greenhouse gas mitigation and negative emissions via advanced biofuels. Proc. Natl Acad. Sci. USA117, 21968–21977 (2020). ArticleCASPubMedPubMed CentralGoogle Scholar
- Baral, N. et al. Techno-economic analysis and life-cycle greenhouse gas mitigation cost of five routes to bio-jet fuel blendstocks. Energy Environ. Sci.12, 807–824 (2018). ArticleGoogle Scholar
- Hannon, J. R. et al. Technoeconomic and life-cycle analysis of single-step catalytic conversion of wet ethanol into fungible fuel blendstocks. Proc. Natl Acad. Sci. USA117, 12576–12583 (2020). ArticleCASPubMedGoogle Scholar
- Baral, N. R. et al. Approaches for more efficient biological conversion of lignocellulosic feedstocks to biofuels and bioproducts. ACS Sustain. Chem. Eng.7, 9062–9079 (2019). ArticleCASGoogle Scholar
- Yang, M., Baral, N. R., Anastasopoulou, A., Breunig, H. M. & Scown, C. D. Cost and life-cycle greenhouse gas implications of integrating biogas upgrading and carbon capture technologies in cellulosic biorefineries. Environ. Sci. Technol.54, 12810–12819 (2020). ArticleCASPubMedGoogle Scholar
- Langholtz, M. H., Stokes, B. J. & Eaton, L. M. Billion-ton report: advancing domestic resources for a thriving bioeconomy, volume 1: economic availability of feedstocks (Oak Ridge National Laboratory, 2016).
- Global Bioenergy Association. Global Bioenergy Statistics 2019. http://www.worldbioenergy.org/uploads/191129%20WBA%20GBS%202019_HQ.pdf (Global Bioenergy Association, 2019).
- Pattrick, C. A. et al. Proteomic profiling, transcription factor modeling, and genomics of evolved tolerant strains elucidate mechanisms of vanillin toxicity in Escherichia coli. mSystems4, e00163-19 (2019). ArticlePubMedPubMed CentralGoogle Scholar
- Carroll, A. & Somerville, C. Cellulosic biofuels. Annu. Rev. Plant Biol.60, 165–182 (2009). ArticleCASPubMedGoogle Scholar
- Blanch, H. W., Simmons, B. A. & Klein-Marcuschamer, D. Biomass deconstruction to sugars. Biotechnol. J.6, 1086–1102 (2011). ArticleCASPubMedGoogle Scholar
- Dale, B. E. & Ong, R. G. Energy, wealth, and human development: why and how biomass pretreatment research must improve. Biotechnol. Prog.28, 893–898 (2012). ArticleCASPubMedGoogle Scholar
- Klein-Marcuschamer, D., Oleskowicz-Popiel, P., Simmons, B. A. & Blanch, H. W. The challenge of enzyme cost in the production of lignocellulosic biofuels. Biotechnol. Bioeng.109, 1083–1087 (2012). ArticleCASPubMedGoogle Scholar
- Lee, S. Y., Kim, H. M. & Cheon, S. Metabolic engineering for the production of hydrocarbon fuels. Curr. Opin. Biotechnol.33, 15–22 (2015). A comprehensive review of metabolic engineering for biofuel production. ArticleCASPubMedGoogle Scholar
- Adom, F., Dunn, J. B., Han, J. & Sather, N. Life-cycle fossil energy consumption and greenhouse gas emissions of bioderived chemicals and their conventional counterparts. Environ. Sci. Technol.48, 14624–14631 (2014). ArticleCASPubMedGoogle Scholar
- Biddy, M. J. et al. The techno-economic basis for coproduct manufacturing to enable hydrocarbon fuel production from lignocellulosic biomass. ACS Sustain. Chem. Eng.4, 3196–3211 (2016). ArticleCASGoogle Scholar
- Brooks, K. P. et al. in Biofuels for Aviation: Feedstocks, Technology and Implementation (ed. Chuck, C.) 109–150 (Academic, 2016).
- George, K. W., Alonso-Gutierrez, J., Keasling, J. D. & Lee, T. S. Isoprenoid drugs, biofuels, and chemicals-artemisinin, farnesene, and beyond. Adv. Biochem. Eng. Biotechnol.148, 355–389 (2015). CASPubMedGoogle Scholar
- Li, M. et al. Recent advances of metabolic engineering strategies in natural isoprenoid production using cell factories. Nat. Prod. Rep.37, 80–99 (2020). ArticleCASPubMedGoogle Scholar
- Rodríguez-Concepción, M. Plant isoprenoids: a general overview. Methods Mol. Biol.1153, 1–5 (2014). ArticlePubMedCASGoogle Scholar
- Gao, Y., Honzatko, R. B. & Peters, R. J. Terpenoid synthase structures: a so far incomplete view of complex catalysis. Nat. Prod. Rep.29, 1153–1175 (2012). ArticleCASPubMedPubMed CentralGoogle Scholar
- Peralta-Yahya, P. P. et al. Identification and microbial production of a terpene-based advanced biofuel. Nat. Commun.2, 483 (2011). ArticlePubMedCASGoogle Scholar
- Harrison, K. W. & Harvey, B. G. Renewable high density fuels containing tricyclic sesquiterpanes and alkyl diamondoids. Sustain. Energy Fuels1, 467–473 (2017). ArticleCASGoogle Scholar
- Zebec, Z. et al. Towards synthesis of monoterpenes and derivatives using synthetic biology. Curr. Opin. Chem. Biol.34, 37–43 (2016). ArticleCASPubMedGoogle Scholar
- George, K. W. et al. Correlation analysis of targeted proteins and metabolites to assess and engineer microbial isopentenol production. Biotechnol. Bioeng.111, 1648–1658 (2014). ArticleCASPubMedGoogle Scholar
- Meadows, A. L. et al. Rewriting yeast central carbon metabolism for industrial isoprenoid production. Nature537, 694–697 (2016). A good demonstration of microbial engineering for biofuel-producing yeast, especially at industrial scale. ArticleCASPubMedGoogle Scholar
- Kang, A. et al. Isopentenyl diphosphate (IPP)-bypass mevalonate pathways for isopentenol production. Metab. Eng.34, 25–35 (2016). ArticleCASPubMedGoogle Scholar
- Kang, A. et al. Optimization of the IPP-bypass mevalonate pathway and fed-batch fermentation for the production of isoprenol in Escherichia coli. Metab. Eng.56, 85–96 (2019). ArticleCASPubMedGoogle Scholar
- Lennen, R. M. & Pfleger, B. F. Engineering Escherichia coli to synthesize free fatty acids. Trends Biotechnol.30, 659–667 (2012). ArticleCASPubMedGoogle Scholar
- Budin, I. et al. Viscous control of cellular respiration by membrane lipid composition. Science362, 1186–1189 (2018). ArticleCASPubMedGoogle Scholar
- Marella, E. R., Holkenbrink, C., Siewers, V. & Borodina, I. Engineering microbial fatty acid metabolism for biofuels and biochemicals. Curr. Opin. Biotechnol.50, 39–46 (2018). ArticleCASPubMedGoogle Scholar
- Qiao, K. et al. Engineering lipid overproduction in the oleaginous yeast Yarrowia lipolytica. Metab. Eng.29, 56–65 (2015). ArticleCASPubMedGoogle Scholar
- Steen, E. J. et al. Microbial production of fatty-acid-derived fuels and chemicals from plant biomass. Nature463, 559–562 (2010). ArticleCASPubMedGoogle Scholar
- Zhou, Y. J. et al. Production of fatty acid-derived oleochemicals and biofuels by synthetic yeast cell factories. Nat. Commun.7, 11709 (2016). ArticleCASPubMedPubMed CentralGoogle Scholar
- Krivoruchko, A., Zhang, Y., Siewers, V., Chen, Y. & Nielsen, J. Microbial acetyl-CoA metabolism and metabolic engineering. Metab. Eng.28, 28–42 (2015). ArticleCASPubMedGoogle Scholar
- Qiao, K., Wasylenko, T. M., Zhou, K., Xu, P. & Stephanopoulos, G. Lipid production in Yarrowia lipolytica is maximized by engineering cytosolic redox metabolism. Nat. Biotechnol.35, 173–177 (2017). ArticleCASPubMedGoogle Scholar
- Zhu, Z. et al. Expanding the product portfolio of fungal type I fatty acid synthases. Nat. Chem. Biol.13, 360–362 (2017). ArticleCASPubMedGoogle Scholar
- Schirmer, A., Rude, M. A., Li, X., Popova, E. & del Cardayre, S. B. Microbial biosynthesis of alkanes. Science329, 559–562 (2010). ArticleCASPubMedGoogle Scholar
- Rude, M. A. et al. Terminal olefin (1-alkene) biosynthesis by a novel p450 fatty acid decarboxylase from Jeotgalicoccus species. Appl. Environ. Microbiol.77, 1718–1727 (2011). ArticleCASPubMedPubMed CentralGoogle Scholar
- Youngquist, J. T. et al. Production of medium chain length fatty alcohols from glucose in Escherichia coli. Metab. Eng.20, 177–186 (2013). ArticleCASPubMedGoogle Scholar
- Goh, E.-B., Baidoo, E. E. K., Keasling, J. D. & Beller, H. R. Engineering of bacterial methyl ketone synthesis for biofuels. Appl. Environ. Microbiol.78, 70–80 (2012). ArticleCASPubMedPubMed CentralGoogle Scholar
- Javidpour, P. et al. Investigation of proposed ladderane biosynthetic genes from anammox bacteria by heterologous expression in E. coli. PLoS ONE11, e0151087 (2016). ArticlePubMedPubMed CentralCASGoogle Scholar
- Czerwiec, Q. et al. Optimization of cyclopropane fatty acids production in Yarrowia lipolytica. Yeast36, 143–151 (2019). CASPubMedGoogle Scholar
- Rabinovitch-Deere, C. A., Oliver, J. W. K., Rodriguez, G. M. & Atsumi, S. Synthetic biology and metabolic engineering approaches to produce biofuels. Chem. Rev.113, 4611–4632 (2013). ArticleCASPubMedGoogle Scholar
- Bond-Watts, B. B., Bellerose, R. J. & Chang, M. C. Y. Enzyme mechanism as a kinetic control element for designing synthetic biofuel pathways. Nat. Chem. Biol.7, 222–227 (2011). ArticleCASPubMedGoogle Scholar
- Atsumi, S., Hanai, T. & Liao, J. C. Non-fermentative pathways for synthesis of branched-chain higher alcohols as biofuels. Nature451, 86–89 (2008). ArticleCASPubMedGoogle Scholar
- Sherkhanov, S. et al. Isobutanol production freed from biological limits using synthetic biochemistry. Nat. Commun.11, 4292 (2020). A good demonstration of the cell-free system for biofuel (isobutanol) production with high TRY. ArticleCASPubMedPubMed CentralGoogle Scholar
- Rodriguez, G. M., Tashiro, Y. & Atsumi, S. Expanding ester biosynthesis in Escherichia coli. Nat. Chem. Biol.10, 259–265 (2014). ArticleCASPubMedPubMed CentralGoogle Scholar
- Lee, J.-W. & Trinh, C. T. Microbial biosynthesis of lactate esters. Biotechnol. Biofuels12, 226 (2019). ArticlePubMedPubMed CentralCASGoogle Scholar
- Yuzawa, S., Keasling, J. D. & Katz, L. Insights into polyketide biosynthesis gained from repurposing antibiotic-producing polyketide synthases to produce fuels and chemicals. J. Antibiot.69, 494–499 (2016). ArticleCASGoogle Scholar
- Cai, W. & Zhang, W. Engineering modular polyketide synthases for production of biofuels and industrial chemicals. Curr. Opin. Biotechnol.50, 32–38 (2018). ArticleCASPubMedGoogle Scholar
- Liu, Q. et al. Engineering an iterative polyketide pathway in Escherichia coli results in single-form alkene and alkane overproduction. Metab. Eng.28, 82–90 (2015). ArticleCASPubMedGoogle Scholar
- Poust, S. et al. Divergent mechanistic routes for the formation of gem-dimethyl groups in the biosynthesis of complex polyketides. Angew. Chem. Int. Ed.54, 2370–2373 (2015). ArticleCASGoogle Scholar
- Srirangan, K. et al. Engineering Escherichia coli for Microbial Production of Butanone. Appl. Environ. Microbiol.82, 2574–2584 (2016). ArticlePubMedPubMed CentralGoogle Scholar
- Yuzawa, S. et al. Short-chain ketone production by engineered polyketide synthases in Streptomyces albus. Nat. Commun.9, 4569 (2018). ArticlePubMedPubMed CentralCASGoogle Scholar
- Zargar, A. et al. Leveraging microbial biosynthetic pathways for the generation of “drop-in” biofuels. Curr. Opin. Biotechnol.45, 156–163 (2017). ArticleCASPubMedPubMed CentralGoogle Scholar
- Smith, K. M., Cho, K.-M. & Liao, J. C. Engineering Corynebacterium glutamicum for isobutanol production. Appl. Microbiol. Biotechnol.87, 1045–1055 (2010). ArticleCASPubMedPubMed CentralGoogle Scholar
- Lin, P. P. et al. Consolidated bioprocessing of cellulose to isobutanol using Clostridium thermocellum. Metab. Eng.31, 44–52 (2015). ArticleCASPubMedGoogle Scholar
- Yan, Q. & Pfleger, B. F. Revisiting metabolic engineering strategies for microbial synthesis of oleochemicals. Metab. Eng.58, 35–46 (2020). ArticleCASPubMedGoogle Scholar
- Hanko, E. K. R. et al. Engineering β-oxidation in Yarrowia lipolytica for methyl ketone production. Metab. Eng.48, 52–62 (2018). ArticleCASPubMedGoogle Scholar
- Kim, H. M., Chae, T. U., Choi, S. Y., Kim, W. J. & Lee, S. Y. Engineering of an oleaginous bacterium for the production of fatty acids and fuels. Nat. Chem. Biol.15, 721–729 (2019). ArticleCASPubMedGoogle Scholar
- Sasaki, Y. et al. Engineering Corynebacterium glutamicum to produce the biogasoline isopentenol from plant biomass hydrolysates. Biotechnol. Biofuels12, 41 (2019). ArticlePubMedPubMed CentralGoogle Scholar
- Yaegashi, J. et al. Rhodosporidium toruloides: a new platform organism for conversion of lignocellulose into terpene biofuels and bioproducts. Biotechnol. Biofuels10, 241 (2017). ArticlePubMedPubMed CentralCASGoogle Scholar
- Sundstrom, E. et al. Demonstrating a separation-free process coupling ionic liquid pretreatment, saccharification, and fermentation with Rhodosporidium toruloides to produce advanced biofuels. Green Chem.20, 2870–2879 (2018). ArticleCASGoogle Scholar
- Zhuang, X. et al. Monoterpene production by the carotenogenic yeast Rhodosporidium toruloides. Microb. Cell Fact.18, 54 (2019). ArticlePubMedPubMed CentralGoogle Scholar
- Miao, R., Xie, H. & Lindblad, P. Enhancement of photosynthetic isobutanol production in engineered cells of Synechocystis PCC 6803. Biotechnol. Biofuels11, 267 (2018). ArticleCASPubMedPubMed CentralGoogle Scholar
- Nguyen, A. D., Kim, D. & Lee, E. Y. Unlocking the biosynthesis of sesquiterpenoids from methane via the methylerythritol phosphate pathway in methanotrophic bacteria, using α-humulene as a model compound. Metab. Eng.61, 69–78 (2020). ArticleCASPubMedGoogle Scholar
- Krieg, T., Sydow, A., Faust, S., Huth, I. & Holtmann, D. CO2 to terpenes: autotrophic and electroautotrophic α-humulene production with Cupriavidus necator. Angew. Chem. Int. Ed.57, 1879–1882 (2018). ArticleCASGoogle Scholar
- Grenz, S. et al. Exploiting Hydrogenophaga pseudoflava for aerobic syngas-based production of chemicals. Metab. Eng.55, 220–230 (2019). ArticleCASPubMedGoogle Scholar
- Mukhopadhyay, A. Tolerance engineering in bacteria for the production of advanced biofuels and chemicals. Trends Microbiol.23, 498–508 (2015). ArticleCASPubMedGoogle Scholar
- Niu, F.-X., He, X., Wu, Y.-Q. & Liu, J.-Z. Enhancing production of pinene in Escherichia coli by using a combination of tolerance, evolution, and modular co-culture engineering. Front. Microbiol.9, 1623 (2018). ArticlePubMedPubMed CentralGoogle Scholar
- Mendez-Perez, D. et al. Production of jet fuel precursor monoterpenoids from engineered Escherichia coli. Biotechnol. Bioeng.114, 1703–1712 (2017). ArticleCASPubMedGoogle Scholar
- Chong, H. et al. Enhancing E. coli isobutanol tolerance through engineering its global transcription factor cAMP receptor protein (CRP). Biotechnol. Bioeng.111, 700–708 (2014). ArticleCASPubMedGoogle Scholar
- Mukhopadhyay, A., Hillson, N. J. & Keasling, J. D. in Microbial Stress Tolerance for Biofuels (ed. Liu, Z. L.) 209–238 (Springer, 2012).
- Park, J. I. et al. A thermophilic ionic liquid-tolerant cellulase cocktail for the production of cellulosic biofuels. PLoS ONE7, e37010 (2012). ArticleCASPubMedPubMed CentralGoogle Scholar
- Yu, C., Simmons, B. A., Singer, S. W., Thelen, M. P. & VanderGheynst, J. S. Ionic liquid-tolerant microorganisms and microbial communities for lignocellulose conversion to bioproducts. Appl. Microbiol. Biotechnol.100, 10237–10249 (2016). ArticleCASPubMedGoogle Scholar
- Thorwall, S., Schwartz, C., Chartron, J. W. & Wheeldon, I. Stress-tolerant non-conventional microbes enable next-generation chemical biosynthesis. Nat. Chem. Biol.16, 113–121 (2020). ArticleCASPubMedGoogle Scholar
- Sandoval, N. R. & Papoutsakis, E. T. Engineering membrane and cell-wall programs for tolerance to toxic chemicals: beyond solo genes. Curr. Opin. Microbiol.33, 56–66 (2016). ArticleCASPubMedPubMed CentralGoogle Scholar
- Basler, G., Thompson, M., Tullman-Ercek, D. & Keasling, J. A Pseudomonas putida efflux pump acts on short-chain alcohols. Biotechnol. Biofuels11, 136 (2018). ArticlePubMedPubMed CentralCASGoogle Scholar
- Chen, B., Ling, H. & Chang, M. W. Transporter engineering for improved tolerance against alkane biofuels in Saccharomyces cerevisiae. Biotechnol. Biofuels6, 21 (2013). ArticleCASPubMedPubMed CentralGoogle Scholar
- Chen, Y. et al. Reverse engineering of fatty acid-tolerant Escherichia coli identifies design strategies for robust microbial cell factories. Metab. Eng.61, 120–130 (2020). ArticleCASPubMedPubMed CentralGoogle Scholar
- Otoupal, P. B. & Chatterjee, A. CRISPR gene perturbations provide insights for improving bacterial biofuel tolerance. Front. Bioeng. Biotechnol.6, 122 (2018). ArticlePubMedPubMed CentralGoogle Scholar
- Kurgan, G. et al. Bioprospecting of native efflux pumps to enhance furfural tolerance in ethanologenic Escherichia coli. Appl. Environ. Microbiol.85, e02985-18 (2019). ArticlePubMedPubMed CentralGoogle Scholar
- Song, H.-S. et al. Increase in furfural tolerance by combinatorial overexpression of NAD salvage pathway enzymes in engineered isobutanol-producing E. coli. Bioresour. Technol.245, 1430–1435 (2017). ArticleCASPubMedGoogle Scholar
- Frederix, M. et al. Development of an E. coli strain for one-pot biofuel production from ionic liquid pretreated cellulose and switchgrass. Green Chem.18, 4189–4197 (2016). ArticleCASGoogle Scholar
- Eng, T. et al. Restoration of biofuel production levels and increased tolerance under ionic liquid stress is enabled by a mutation in the essential Escherichia coli gene cydC. Microb. Cell Fact.17, 159 (2018). ArticleCASPubMedPubMed CentralGoogle Scholar
- Ruegg, T. L. et al. Jungle Express is a versatile repressor system for tight transcriptional control. Nat. Commun.9, 3617 (2018). ArticlePubMedPubMed CentralCASGoogle Scholar
- Wang, S. et al. NaCl enhances Escherichia coli growth and isoprenol production in the presence of imidazolium-based ionic liquids. Bioresour. Technol. Rep.6, 1–5 (2019). ArticleGoogle Scholar
- Nikel, P. I. & de Lorenzo, V. Pseudomonas putida as a functional chassis for industrial biocatalysis: from native biochemistry to trans-metabolism. Metab. Eng.50, 142–155 (2018). ArticleCASPubMedGoogle Scholar
- Yang, S. et al. Zymomonas mobilis as a model system for production of biofuels and biochemicals. Microb. Biotechnol.9, 699–717 (2016). ArticleCASPubMedPubMed CentralGoogle Scholar
- Stella, R. G., Wiechert, J., Noack, S. & Frunzke, J. Evolutionary engineering of Corynebacterium glutamicum. Biotechnol. J.14, e1800444 (2019). ArticlePubMedCASGoogle Scholar
- Castro, A. R., Rocha, I., Alves, M. M. & Pereira, M. A. Rhodococcus opacus B4: a promising bacterium for production of biofuels and biobased chemicals. AMB. Express6, 35 (2016). ArticlePubMedPubMed CentralCASGoogle Scholar
- Thompson, M. G. et al. Fatty acid and alcohol metabolism in Pseudomonas putida: functional analysis using random barcode transposon sequencing. Appl. Environ. Microbiol.86, e01665-20 (2020). ArticlePubMedPubMed CentralGoogle Scholar
- Sandberg, T. E., Salazar, M. J., Weng, L. L., Palsson B. O. & Feist, A. M. The emergence of adaptive laboratory evolution as an efficient tool for biological discovery and industrial biotechnology. Metab. Eng.56, 1–16 (2019). ArticleCASPubMedPubMed CentralGoogle Scholar
- Lim, H. G. et al. Generation of ionic liquid tolerant Pseudomonas putida KT2440 strains via adaptive laboratory evolution. Green Chem.22, 5677–5690 (2020). ArticleCASGoogle Scholar
- Price, M. N. et al. Mutant phenotypes for thousands of bacterial genes of unknown function. Nature557, 503–509 (2018). A study showing the power of the RB-TnSeq approach in elucidating gene function in a vast number of microorganisms, which is not only valuable for identifying gene targets for strain engineering but is also broadly useful for improving GSMMs. ArticleCASPubMedGoogle Scholar
- Li, W.-J. et al. Unraveling 1,4-butanediol metabolism in Pseudomonas putida KT2440. Front. Microbiol.11, 382 (2020). ArticlePubMedPubMed CentralGoogle Scholar
- Phaneuf, P. V., Gosting, D., Palsson, B. O. & Feist, A. M. ALEdb 1.0: a database of mutations from adaptive laboratory evolution experimentation. Nucleic Acids Res.47, D1164–D1171 (2019). ArticlePubMedGoogle Scholar
- Zhang, F., Carothers, J. M. & Keasling, J. D. Design of a dynamic sensor-regulator system for production of chemicals and fuels derived from fatty acids. Nat. Biotechnol.30, 354–359 (2012). ArticleCASPubMedGoogle Scholar
- DeLoache, W. C., Russ, Z. N. & Dueber, J. E. Towards repurposing the yeast peroxisome for compartmentalizing heterologous metabolic pathways. Nat. Commun.7, 11152 (2016). ArticleCASPubMedPubMed CentralGoogle Scholar
- Hu, T. et al. Engineering chimeric diterpene synthases and isoprenoid biosynthetic pathways enables high-level production of miltiradiene in yeast. Metab. Eng.60, 87–96 (2020). ArticleCASPubMedGoogle Scholar
- McCloskey, D. et al. Adaptation to the coupling of glycolysis to toxic methylglyoxal production in tpiA deletion strains of Escherichia coli requires synchronized and counterintuitive genetic changes. Metab. Eng.48, 82–93 (2018). ArticleCASPubMedGoogle Scholar
- Gubellini, F. et al. Physiological response to membrane protein overexpression in E. coli. Mol. Cell. Proteom.10, M111.007930 (2011). ArticleCASGoogle Scholar
- Baumgarten, T., Ytterberg, A. J., Zubarev, R. A. & de Gier, J.-W. Optimizing recombinant protein production in the Escherichia coli periplasm alleviates stress. Appl. Environ. Microbiol.84, e00270-18 (2018). ArticlePubMedPubMed CentralGoogle Scholar
- Boyarskiy, S., Davis López, S., Kong, N. & Tullman-Ercek, D. Transcriptional feedback regulation of efflux protein expression for increased tolerance to and production of n-butanol. Metab. Eng.33, 130–137 (2016). ArticleCASPubMedGoogle Scholar
- Henard, C. A., Freed, E. F. & Guarnieri, M. T. Phosphoketolase pathway engineering for carbon-efficient biocatalysis. Curr. Opin. Biotechnol.36, 183–188 (2015). ArticleCASPubMedGoogle Scholar
- Lin, P. P. et al. Construction and evolution of an Escherichia coli strain relying on nonoxidative glycolysis for sugar catabolism. Proc. Natl Acad. Sci. USA115, 3538–3546 (2018). A very detailed and extensive demonstration of engineering non-oxidative glycolysis. ArticleCASPubMedPubMed CentralGoogle Scholar
- Bogorad, I. W., Lin, T.-S. & Liao, J. C. Synthetic non-oxidative glycolysis enables complete carbon conservation. Nature502, 693–697 (2013). ArticleCASPubMedGoogle Scholar
- Fleige, C., Kroll, J. & Steinbüchel, A. Establishment of an alternative phosphoketolase-dependent pathway for fructose catabolism in Ralstonia eutropha H16. Appl. Microbiol. Biotechnol.91, 769–776 (2011). ArticleCASPubMedGoogle Scholar
- Chubukov, V., Mukhopadhyay, A., Petzold, C. J., Keasling, J. D. & Martín, H. G. Synthetic and systems biology for microbial production of commodity chemicals. NPJ Syst. Biol. Appl.2, 16009 (2016). ArticleCASPubMedPubMed CentralGoogle Scholar
- Tian, T., Kang, J. W., Kang, A. & Lee, T. S. Redirecting metabolic flux via combinatorial multiplex CRISPRi-mediated repression for isopentenol production in Escherichia coli. ACS Synth. Biol.8, 391–402 (2019). ArticleCASPubMedGoogle Scholar
- George, K. W. et al. Metabolic engineering for the high-yield production of isoprenoid-based C5 alcohols in E. coli. Sci. Rep.5, 11128 (2015). ArticlePubMedPubMed CentralGoogle Scholar
- Strucko, T. et al. Laboratory evolution reveals regulatory and metabolic trade-offs of glycerol utilization in Saccharomyces cerevisiae. Metab. Eng.47, 73–82 (2018). ArticleCASPubMedGoogle Scholar
- Caspeta, L. et al. Biofuels. Altered sterol composition renders yeast thermotolerant. Science346, 75–78 (2014). ArticleCASPubMedGoogle Scholar
- Mohamed, E. T. et al. Generation of a platform strain for ionic liquid tolerance using adaptive laboratory evolution. Microb. Cell Fact.16, 204 (2017). ArticlePubMedPubMed CentralCASGoogle Scholar
- Lennen, R. M. et al. Adaptive laboratory evolution reveals general and specific chemical tolerance mechanisms and enhances biochemical production. Preprint at bioRxivhttps://doi.org/10.1101/634105 (2019). ArticleGoogle Scholar
- Shepelin, D., Hansen, A. S. L., Lennen, R., Luo, H. & Herrgård, M. J. Selecting the best: evolutionary engineering of chemical production in microbes. Genes9, 249 (2018). An excellent review on growth coupling. ArticlePubMed CentralCASGoogle Scholar
- Fong, S. S. et al. In silico design and adaptive evolution of Escherichia coli for production of lactic acid. Biotechnol. Bioeng.91, 643–648 (2005). ArticleCASPubMedGoogle Scholar
- Zhang, X., Jantama, K., Moore, J. C., Shanmugam, K. T. & Ingram, L. O. Production of L-alanine by metabolically engineered Escherichia coli. Appl. Microbiol. Biotechnol.77, 355–366 (2007). ArticleCASPubMedGoogle Scholar
- Shen, C. R. et al. Driving forces enable high-titer anaerobic 1-butanol synthesis in Escherichia coli. Appl. Environ. Microbiol.77, 2905–2915 (2011). ArticleCASPubMedPubMed CentralGoogle Scholar
- Machado, H. B., Dekishima, Y., Luo, H., Lan, E. I. & Liao, J. C. A selection platform for carbon chain elongation using the CoA-dependent pathway to produce linear higher alcohols. Metab. Eng.14, 504–511 (2012). ArticleCASPubMedGoogle Scholar
- Reyes, L. H., Gomez, J. M. & Kao, K. C. Improving carotenoids production in yeast via adaptive laboratory evolution. Metab. Eng.21, 26–33 (2014). ArticleCASPubMedGoogle Scholar
- Tai, Y.-S. et al. Engineering nonphosphorylative metabolism to generate lignocellulose-derived products. Nat. Chem. Biol.12, 247–253 (2016). ArticleCASPubMedGoogle Scholar
- Hädicke, O. & Klamt, S. Computing complex metabolic intervention strategies using constrained minimal cut sets. Metab. Eng.13, 204–213 (2011). ArticlePubMedCASGoogle Scholar
- Harder, B.-J., Bettenbrock, K. & Klamt, S. Model-based metabolic engineering enables high yield itaconic acid production by Escherichia coli. Metab. Eng.38, 29–37 (2016). ArticleCASPubMedGoogle Scholar
- von Kamp, A. & Klamt, S. Growth-coupled overproduction is feasible for almost all metabolites in five major production organisms. Nat. Commun.8, 15956 (2017). ArticleCASGoogle Scholar
- Banerjee, D. et al. Genome-scale metabolic rewiring improves titers rates and yields of the non-native product indigoidine at scale. Nat. Commun.11, 5385 (2020). ArticleCASPubMedPubMed CentralGoogle Scholar
- King, Z. A. et al. BiGG models: a platform for integrating, standardizing and sharing genome-scale models. Nucleic Acids Res.44, D515–D522 (2016). ArticleCASPubMedGoogle Scholar
- Landon, S., Rees-Garbutt, J., Marucci, L. & Grierson, C. Genome-driven cell engineering review: in vivo and in silico metabolic and genome engineering. Essays Biochem.63, 267–284 (2019). ArticleCASPubMedPubMed CentralGoogle Scholar
- Yim, H. et al. Metabolic engineering of Escherichia coli for direct production of 1,4-butanediol. Nat. Chem. Biol.7, 445–452 (2011). ArticleCASPubMedGoogle Scholar
- Ng, C. Y., Jung, M.-Y., Lee, J. & Oh, M.-K. Production of 2,3-butanediol in Saccharomyces cerevisiae by in silico aided metabolic engineering. Microb. Cell Fact.11, 68 (2012). ArticleCASPubMedPubMed CentralGoogle Scholar
- Izallalen, M. et al. Geobacter sulfurreducens strain engineered for increased rates of respiration. Metab. Eng.10, 267–275 (2008). ArticleCASPubMedGoogle Scholar
- Fowler, Z. L., Gikandi, W. W. & Koffas, M. A. G. Increased malonyl coenzyme A biosynthesis by tuning the Escherichia coli metabolic network and its application to flavanone production. Appl. Environ. Microbiol.75, 5831–5839 (2009). ArticleCASPubMedPubMed CentralGoogle Scholar
- Chemler, J. A., Fowler, Z. L., McHugh, K. P. & Koffas, M. A. G. Improving NADPH availability for natural product biosynthesis in Escherichia coli by metabolic engineering. Metab. Eng.12, 96–104 (2010). ArticleCASPubMedGoogle Scholar
- Asadollahi, M. A. et al. Enhancing sesquiterpene production in Saccharomyces cerevisiae through in silico driven metabolic engineering. Metab. Eng.11, 328–334 (2009). ArticleCASPubMedGoogle Scholar
- Brochado, A. R. et al. Improved vanillin production in baker’s yeast through in silico design. Microb. Cell Fact.9, 84 (2010). ArticlePubMedPubMed CentralCASGoogle Scholar
- Choi, H. S., Lee, S. Y., Kim, T. Y. & Woo, H. M. In silico identification of gene amplification targets for improvement of lycopene production. Appl. Environ. Microbiol.76, 3097–3105 (2010). ArticleCASPubMedPubMed CentralGoogle Scholar
- Becker, J., Zelder, O., Häfner, S., Schröder, H. & Wittmann, C. From zero to hero–design-based systems metabolic engineering of Corynebacterium glutamicum for L-lysine production. Metab. Eng.13, 159–168 (2011). ArticleCASPubMedGoogle Scholar
- Li, S., Huang, D., Li, Y., Wen, J. & Jia, X. Rational improvement of the engineered isobutanol-producing Bacillus subtilis by elementary mode analysis. Microb. Cell Fact.11, 101 (2012). ArticleCASPubMedPubMed CentralGoogle Scholar
- Xu, P., Ranganathan, S., Fowler, Z. L., Maranas, C. D. & Koffas, M. A. G. Genome-scale metabolic network modeling results in minimal interventions that cooperatively force carbon flux towards malonyl-CoA. Metab. Eng.13, 578–587 (2011). ArticleCASPubMedGoogle Scholar
- Ranganathan, S. et al. An integrated computational and experimental study for overproducing fatty acids in Escherichia coli. Metab. Eng.14, 687–704 (2012). An illustrative example of using GSMMs to guide bioengineering. ArticleCASPubMedGoogle Scholar
- Otero, J. M. et al. Industrial systems biology of Saccharomyces cerevisiae enables novel succinic acid cell factory. PLoS ONE8, e54144 (2013). ArticleCASPubMedPubMed CentralGoogle Scholar
- Ghosh, A. et al. 13 C metabolic flux analysis for systematic metabolic engineering of S. cerevisiae for overproduction of fatty acids. Front. Bioeng. Biotechnol.4, 76 (2016). ArticlePubMedPubMed CentralGoogle Scholar
- d’Espaux, L. et al. Engineering high-level production of fatty alcohols by Saccharomyces cerevisiae from lignocellulosic feedstocks. Metab. Eng.42, 115–125 (2017). ArticlePubMedCASGoogle Scholar
- Lawson, C. E. et al. Machine learning for metabolic engineering: a review. Metab. Eng. 63, 34–60. A good introduction to machine learning for the metabolic engineer.
- Alonso-Gutierrez, J. et al. Principal component analysis of proteomics (PCAP) as a tool to direct metabolic engineering. Metab. Eng.28, 123–133 (2015). ArticleCASPubMedGoogle Scholar
- Ohtake, T. et al. Metabolomics-driven approach to solving a CoA imbalance for improved 1-butanol production in Escherichia coli. Metab. Eng.41, 135–143 (2017). ArticleCASPubMedGoogle Scholar
- Xu, P., Rizzoni, E. A., Sul, S.-Y. & Stephanopoulos, G. Improving metabolic pathway efficiency by statistical model-based multivariate regulatory metabolic engineering. ACS Synth. Biol.6, 148–158 (2017). ArticleCASPubMedGoogle Scholar
- Zhou, Y. et al. MiYA, an efficient machine-learning workflow in conjunction with the YeastFab assembly strategy for combinatorial optimization of heterologous metabolic pathways in Saccharomyces cerevisiae. Metab. Eng.47, 294–302 (2018). ArticleCASPubMedGoogle Scholar
- Costello, Z. & Martin, H. G. A machine learning approach to predict metabolic pathway dynamics from time-series multiomics data. NPJ Syst. Biol. Appl.4, 19 (2018). ArticlePubMedPubMed CentralGoogle Scholar
- Opgenorth, P. et al. Lessons from two design-build-test-learn cycles of dodecanol production in Escherichia coli aided by machine learning. ACS Synth. Biol.8, 1337–1351 (2019). ArticleCASPubMedGoogle Scholar
- Jervis, A. J. et al. Machine learning of designed translational control allows predictive pathway optimization in Escherichia coli. ACS Synth. Biol.8, 127–136 (2019). An excellent application of machine learning to transcriptional control. ArticleCASPubMedGoogle Scholar
- HamediRad, M. et al. Towards a fully automated algorithm driven platform for biosystems design. Nat. Commun.10, 5150 (2019). A fantastic example of the promise of combining machine learning, synthetic biology and automation. ArticlePubMedPubMed CentralCASGoogle Scholar
- Zhang, J. et al. Combining mechanistic and machine learning models for predictive engineering and optimization of tryptophan metabolism. Nat. Commun.11, 880 (2020). CASGoogle Scholar
- Radivojević, T., Costello, Z., Workman, K. & Garcia Martin, H. A machine learning automated recommendation tool for synthetic biology. Nat. Commun.11, 4879 (2020). ArticlePubMedPubMed CentralCASGoogle Scholar
- Yadav, V. G., De Mey, M., Lim, C. G., Ajikumar, P. K. & Stephanopoulos, G. The future of metabolic engineering and synthetic biology: towards a systematic practice. Metab. Eng.14, 233–241 (2012). ArticleCASPubMedPubMed CentralGoogle Scholar
- Carbonell, P., Radivojevic, T. & García Martín, H. Opportunities at the intersection of synthetic biology, machine learning, and automation. ACS Synth. Biol.8, 1474–1477 (2019). ArticleCASPubMedGoogle Scholar
- Dietrich, J. A., McKee, A. E. & Keasling, J. D. High-throughput metabolic engineering: advances in small-molecule screening and selection. Annu. Rev. Biochem.79, 563–590 (2010). ArticleCASPubMedGoogle Scholar
- Crater, J. S. & Lievense, J. C. Scale-up of industrial microbial processes. FEMS Microbiol. Lett.365, fny138 (2018). ArticleCASPubMed CentralGoogle Scholar
- Davis, R. et al. Process design and economics for the conversion of lignocellulosic biomass to hydrocarbons: dilute-acid and enzymatic deconstruction of biomass to sugars and biological conversion of sugars to hydrocarbons (National Renewable Energy Laboratory, 2013).
- Cruz Bournazou, M. N. et al. Online optimal experimental re-design in robotic parallel fed-batch cultivation facilities. Biotechnol. Bioeng.114, 610–619 (2017). ArticleCASPubMedGoogle Scholar
- Tai, M., Ly, A., Leung, I. & Nayar, G. Efficient high-throughput biological process characterization: definitive screening design with the Ambr250 bioreactor system. Biotechnol. Prog.31, 1388–1395 (2015). ArticleCASPubMedGoogle Scholar
- Wong, B. G., Mancuso, C. P., Kiriakov, S., Bashor, C. J. & Khalil, A. S. Precise, automated control of conditions for high-throughput growth of yeast and bacteria with eVOLVER. Nat. Biotechnol.36, 614–623 (2018). ArticleCASPubMedPubMed CentralGoogle Scholar
- Haringa, C. et al. Computational fluid dynamics simulation of an industrial P. chrysogenum fermentation with a coupled 9-pool metabolic model: towards rational scale-down and design optimization. Chem. Eng. Sci.175, 12–24 (2017). ArticleCASGoogle Scholar
- Rugbjerg, P. & Sommer, M. O. A. Overcoming genetic heterogeneity in industrial fermentations. Nat. Biotechnol.37, 869–876 (2019). ArticleCASPubMedGoogle Scholar
- Wehrs, M. et al. Investigation of Bar-seq as a method to study population dynamics of Saccharomyces cerevisiae deletion library during bioreactor cultivation. Microb. Cell Fact.19, 167 (2020). ArticleCASPubMedPubMed CentralGoogle Scholar
- Wang, O. & Coates, J. D. Biotechnological applications of microbial (per)chlorate reduction. Microorganisms5, 76 (2017). ArticleCASPubMed CentralGoogle Scholar
- Shaw, A. J. et al. Metabolic engineering of microbial competitive advantage for industrial fermentation processes. Science353, 583–586 (2016). ArticleCASPubMedGoogle Scholar
- Dahl, R. H. et al. Engineering dynamic pathway regulation using stress-response promoters. Nat. Biotechnol.31, 1039–1046 (2013). ArticleCASPubMedGoogle Scholar
- Dafoe, J. T. & Daugulis, A. J. In situ product removal in fermentation systems: improved process performance and rational extractant selection. Biotechnol. Lett.36, 443–460 (2014). ArticleCASPubMedGoogle Scholar
- Xue, C. et al. Integrated butanol recovery for an advanced biofuel: current state and prospects. Appl. Microbiol. Biotechnol.98, 3463–3474 (2014). ArticleCASPubMedGoogle Scholar
- Gaspar, D. Top ten blendstocks for turbocharged gasoline engines: bioblendstocks with potential to deliver the for highest engine efficiency (Pacific Northwest National Laboratory, 2019). A systematic analysis and down-election of petrol bioblendstock candidates based on fuel properties and engine performance.
- Monroe, E. et al. Discovery of novel octane hyperboosting phenomenon in prenol biofuel/gasoline blends. Fuel239, 1143–1148 (2019). ArticleCASGoogle Scholar
- Ignea, C. et al. Synthesis of 11-carbon terpenoids in yeast using protein and metabolic engineering. Nat. Chem. Biol.14, 1090–1098 (2018). ArticleCASPubMedGoogle Scholar
- Huccetogullari, D., Luo, Z. W. & Lee, S. Y. Metabolic engineering of microorganisms for production of aromatic compounds. Microb. Cell Fact.18, 41 (2019). ArticlePubMedPubMed CentralGoogle Scholar
- Das, D. D., St. John, P. C., McEnally, C. S., Kim, S. & Pfefferle, L. D. Measuring and predicting sooting tendencies of oxygenates, alkanes, alkenes, cycloalkanes, and aromatics on a unified scale. Combust. Flame190, 349–364 (2018). ArticleCASGoogle Scholar
- Huo, X. et al. Tailoring diesel bioblendstock from integrated catalytic upgrading of carboxylic acids: a “fuel property first” approach. Green Chem.21, 5813–5827 (2019). ArticleCASGoogle Scholar
- Yang, M. et al. Accumulation of high-value bioproducts in planta can improve the economics of advanced biofuels. Proc. Natl Acad. Sci. USA117, 8639–8648 (2020). ArticleCASPubMedPubMed CentralGoogle Scholar
- Lin, C.-Y. & Eudes, A. Strategies for the production of biochemicals in bioenergy crops. Biotechnol. Biofuels13, 71 (2020). ArticlePubMedPubMed CentralGoogle Scholar
- Blombach, B. et al. Corynebacterium glutamicum tailored for efficient isobutanol production. Appl. Environ. Microbiol.77, 3300–3310 (2011). ArticleCASPubMedPubMed CentralGoogle Scholar
- Higashide, W., Li, Y., Yang, Y. & Liao, J. C. Metabolic engineering of Clostridium cellulolyticum for production of isobutanol from cellulose. Appl. Environ. Microbiol.77, 2727–2733 (2011). ArticleCASPubMedPubMed CentralGoogle Scholar
- Sawyer, R. F. Trends in auto emissions and gasoline composition. Environ. Health Perspect.101 (Suppl. 6), 5–12 (1993). ArticleCASPubMedPubMed CentralGoogle Scholar
- Ghosh, P., Hickey, K. J. & Jaffe, S. B. Development of a detailed gasoline composition-based octane model. Ind. Eng. Chem. Res.45, 337–345 (2006). ArticleCASGoogle Scholar
- Ghosh, P. & Jaffe, S. B. Detailed composition-based model for predicting the cetane number of diesel fuels. Ind. Eng. Chem. Res.45, 346–351 (2006). ArticleCASGoogle Scholar
- ASTM International. ASTM D1655 — 20e1: standard specification for aviation turbine fuels (ASTM, 2020).
Acknowledgements
The authors thank C. Scown (Lawrence Berkeley National Laboratory) for helpful discussions on life cycle and technoeconomic analyses of biofuel production. This work was performed as part of the US Department of Energy (DOE) Joint BioEnergy Institute (https://www.jbei.org) supported by the DOE, Office of Science, Office of Biological and Environmental Research, and by the DOE, Energy Efficiency and Renewable Energy, Bioenergy Technologies Office, and as part of the Co-Optimization of Fuels & Engines project sponsored by the DOE, Office of Energy Efficiency and Renewable Energy, Bioenergy Technologies Office and Vehicle Technologies Office, under contract DEAC02-05CH11231 between the DOE and Lawrence Berkeley National Laboratory. The views and opinions of the authors expressed herein do not necessarily state or reflect those of the US Government or any agency thereof. Neither the US Government nor any agency thereof, nor any of their employees, makes any warranty, expressed or implied, or assumes any legal liability or responsibility for the accuracy, completeness or usefulness of any information, apparatus, product or process disclosed, or represents that its use would not infringe privately owned rights. The US Government retains and the publisher, by accepting the article for publication, acknowledges that the US Government retains a non-exclusive, paid-up, irrevocable, worldwide license to publish or reproduce the published form of the manuscript, or allow others to do so, for US Government purposes. The DOE will provide public access to these results of federally sponsored research in accordance with the DOE Public Access Plan (http://energy.gov/downloads/doe-public-access-plan).
Author information
Authors and Affiliations
- Joint BioEnergy Institute, Emeryville, CA, USA Jay Keasling, Hector Garcia Martin, Taek Soon Lee, Aindrila Mukhopadhyay & Steven W. Singer
- Department of Chemical & Biomolecular Engineering, University of California, Berkeley, Berkeley, CA, USA Jay Keasling
- Department of Bioengineering, University of California, Berkeley, Berkeley, CA, USA Jay Keasling
- Biological Systems and Engineering Division, Lawrence Berkeley National Laboratory, Berkeley, CA, USA Jay Keasling, Hector Garcia Martin, Taek Soon Lee, Aindrila Mukhopadhyay, Steven W. Singer & Eric Sundstrom
- Center for Biosustainability, Danish Technical University, Lyngby, Denmark Jay Keasling
- Center for Synthetic Biochemistry, Institute for Synthetic Biology, Shenzhen Institute of Advanced Technology, Shenzhen, China Jay Keasling
- DOE Agile BioFoundry, Emeryville, CA, USA Hector Garcia Martin
- BCAM,Basque Center for Applied Mathematics, Bilbao, Spain Hector Garcia Martin
- Environmental Genomics and Systems Biology Division, Lawrence Berkeley National Laboratory, Berkeley, CA, USA Hector Garcia Martin & Aindrila Mukhopadhyay
- Advanced Biofuels and Bioproducts Process Development Unit, Emeryville, CA, USA Eric Sundstrom
- Jay Keasling